Technology is taking place everywhere in the world. It plays a significant role in every industry and works wonders, including machine learning, engineering, and many more. Linear and non-linear machine optimizations also help with various problems and make them easy. Non-linear machine optimization is one of the crucial areas of studies that help in solving complex issues. Both linear and non-linear machine optimizations have their own benefits and workings.
If you are confused about non-linear machine optimization and its benefits, this guide is for you. Here, we will explain non-linear machine optimization along with all its significant aspects.
What Is Non-Linear Machine Optimization?
Non-linear machine optimization is significantly involved in finding solutions where constraints are non-linear, and the relationship between two variables is complex. It will simply bring the linearity in variables and make the issue simple by including quadratic and exponential functions.
The primary goal of non-linear machine optimization is to minimize and elevate the objective function. It represents anything in a business, including cost, energy, and error rates that involve machine learning models. However, there also comes a challenge in that the non-linear functions have different local minimums and maximums, which makes it difficult to find the optimum values.
The non-linear machine optimizations include objective functions, variables, constraints, and optimization algorithms.
Examples of Non-Linear Machine Optimization
Examples of Non-Linear Machine Optimization
Non-linear machine optimization involves different examples. However, its most basic use is in neural networks, supply chain or logistics, and portfolio optimization.
In neural networks, it trains the network to minimize the non-linear loss functions and optimize the model accuracy. The algorithms are crucial in adjusting the networks and reducing prediction errors.
Non-linear machine optimization helps supply chain businesses optimize their scheduling, routing, and resourcing. You can take account of real-world nonlinearities, including fuel, and make fluctuating demands accordingly.
Benefits of Non-Linear Machine Optimization
Linear and non-linear machine optimization have their own benefits. The non-linear module offers a wide range of benefits, especially when it comes to complex problems and linear models are not working effectively.
Solve Complex Real-World Problems
We all have issues that involve non-linear relationships rather than simply linear models. Non-linear models can optimize complex issues more accurately and effectively, including engineering and physics processes. They optimize robotic vehicles and similar models that include non-linear equations. They ensure that all these systems work effectively, even under various constraints.
Increased Flexibility and Applicability
Many global industries that work with management and logistics significantly benefit from non-linear machine optimization. It helps improve resource allocation and reduce energy consumption. Moreover, it enhances operational efficiency and reduces fuel costs in transportation. So, well-optimized vehicle routes in real-time data, weather conditions, and traffic patterns will help logistic companies gain better profit.
Non-linear optimization also helps improve the production line efficiently and minimize downtime.
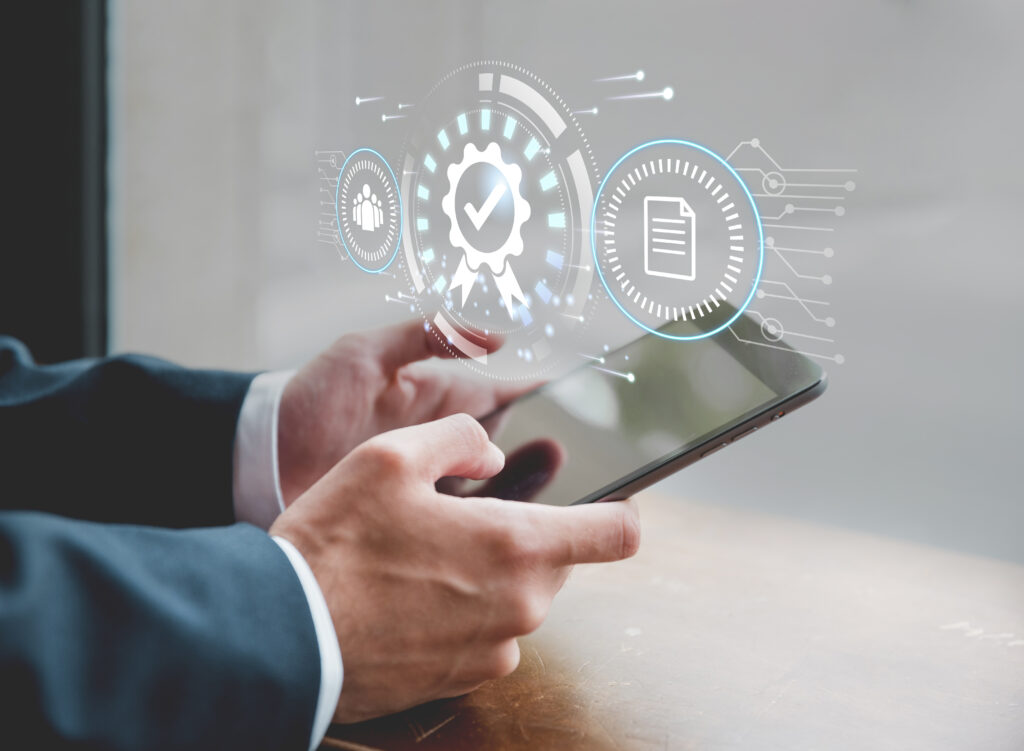
Better Resource Management
Non-linear optimization helps in optimizing resource allocation in various industries, including agriculture, energy, and water management. It can analyze complex resources and their usage and optimize algorithms and purpose solutions according to them.
Integration With Other Tools
Taskade will effectively integrate with other third-party tools, including Google Calendar and Dropbox. It will ensure your data is safe and synced across the platforms. You can enjoy a better workflow without needing AI tools.
Challenges of Non-Linear Optimization
Non-linear optimization has plenty of benefits, but it also has some challenges.
Non-linear problems often demand more computationally complex algorithms than linear problems. Moreover, non-linear machine optimization, like gradients, can be sensitive and require more careful selection from the start. This will help avoid poor solutions in the end.
So, non-linear machine optimization is essential for solving real-world complex issues where linear optimization does not work.
Ready to revolutionize your marketing strategy with AI?
Visit Cellit Online and explore more about the future of AI in marketing, its transformative benefits, and how you can leverage this technology for your business growth! Read our in-depth blogs:
- AI in Online Shopping: Transforming How We Buy and Sell
- AI-Powered Ad Management: Revolutionizing Digital Marketing
- AI Transformation: Key Benefits and Challenges for Businesses
- How AI Ad Generators Can Save Time
- Why Video Marketing is So Powerful
- How to Create Different Video Ad Variation: A Comprehensive Guide
- How to Train Video Generation AI
- Which of the following is generative AI application?
- The Importance of Seamless Communication: Essential Tips to Follow
- How Long can a Video be on Messaging Apps?
Don’t miss out on the AI revolution!